Optimization Learning And Natural Algorithms Phd Thesis. with separate individual learning or local. Ant colony optimization algorithms have been applied to many combinatorial optimization problems. Learning and Natural Algorithms, PhD thesis. Optimization, Learning and Natural Algorithms.” blogger.comted Reading Time: 3 mins Our Ant Colony Optimization Phd Thesis online essay writing service delivers Master’s level writing by experts who have earned graduate degrees in your subject Ant Colony Optimization Phd Thesis matter. All citations and writing are % original. Your thesis is /10() Training feed-forward neural networks with ant colony optimization: An application to pattern classification. In Hybrid Intelligent Systems, HIS’ Fifth International Conference on, pages 6–pp. IEEE, 4. A. Pandian. Training neural networks with ant colony optimization. PhD thesis, California State University, Sacramento, 5. M
Marco dorigo phd thesis proposal
Ant colony optimization ACO is a probabalistic stochasticheuristic optimization technique inspired by the way ants make and find paths from the colony to food. The technique is used to solve discrete optimization problems that can be reduced to finding good paths through graphs. The first appearance of an ACO system was in a Ph. thesis in by Marco Dorigo at Politecnico di Milano. It was called Ant System AS.
Since various other extended versions of AS have been developed, including Ant Colony System ACS and MAX-MIN Ant System MMAS, ant colony optimization phd thesis. In Dorigo proposed the Ant Colony Optimization ACO metaheuristic that became the most successful and recognized algorithm based on ant behavior, ant colony optimization phd thesis.
Ant colony optimization phd thesis searching for food, ants will wander around randomly until they find a food source, and then return to the colony while laying down a pheromone path that can be retraced by other ants.
The ants at the colony that are wandering around will pick up this trail and follow it to the food and on the return to the colony they will also reinforce the trail by laying down a pheromone path. The pheromone path is not permanent and evaporates over time. This means that the paths that are longer will be weaker since there is more time between pheromone dropping. However, a ant colony optimization phd thesis path will have a much stronger pheromone path since it is closer and will be replenished with pheromones continuously.
Thus the shorter paths will be preferred and the ants will tend to travel to the optimum closest food source. When one ant finds an optimal path from the colony to a food source, other ants are more likely to follow that path and positive feedback eventually causes all the ants to follow the same path.
The ACO algorithm mimics this by walking around the graph representing the problem to solve. These algorithms have been applied to the symmetric and asymmetric traveling salesman problem with near-optimal results.
There has been an interest in using ACO for network routing and urban transportation systems since the algorithm can be run continuously giving it the ability to adapt to changes in real time. This is an advantage over the simulated annealing and genetic algorithm approaches since they do not change dynamically. In machine learning and data mining problems, ACO variations have been used to create a model of the way worker ants "cluster" ant corpses in ant cemetary maintenance.
This has been applied to a task called clustering in machine learning which involves finding groups ant colony optimization phd thesis objects that are similar. This method has proven to have higher performance and accuracy than previous classical methods.
Ant colony optimization From DDL Wiki. Jump to: navigationsearch. Category : Optimization. Views Page Discussion View source History. Personal tools Log in. Navigation Main Page Community portal Current events Recent changes Random page Help. Toolbox What links here Related changes Upload file Special pages Printable version Permanent link. Contents 1 History 2 Real World Ant Behavior 3 Application 4 External Links 5 References.
Ant Colony Optimization Simulation
, time: 3:19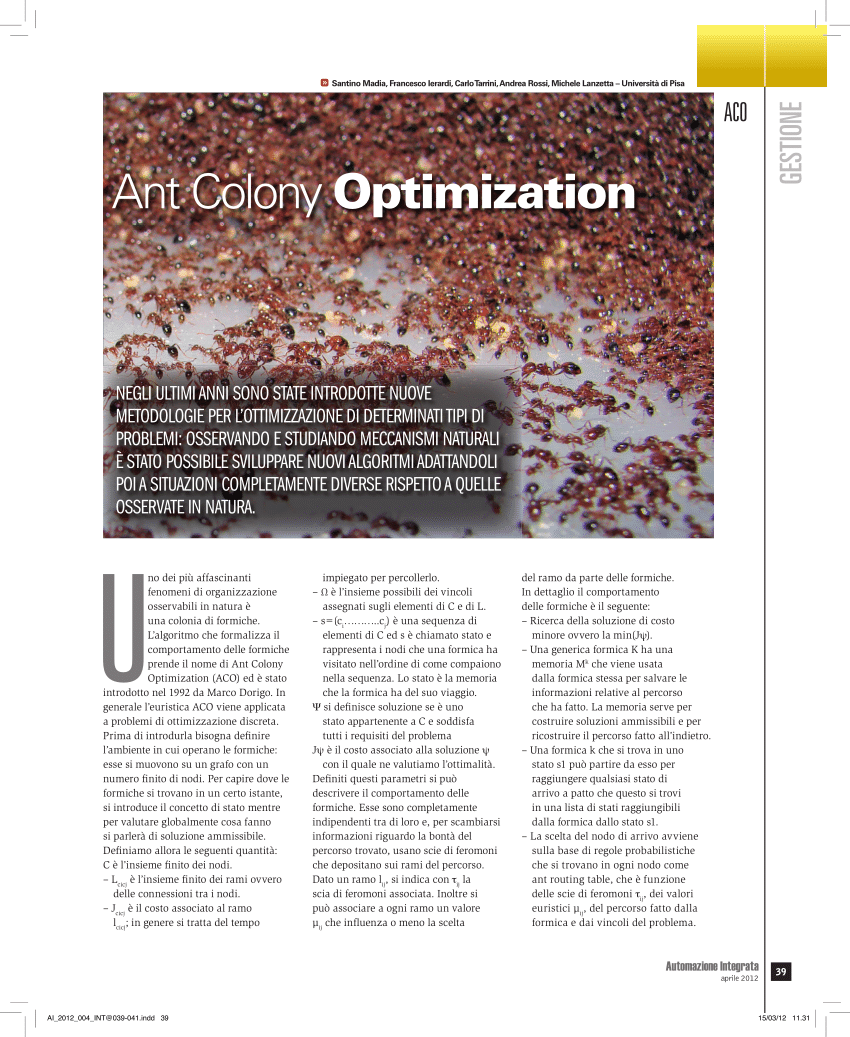
In this dissertation, I will report our research work on constructing new heuristic algorithms using the Ant Colony metaheuristic for effectively and efficiently solving a range of difficult architectural design problems. We invest igate three N P-hard prob-lems in this context, namely system partitioning, operation scheduling and design space Optimization Learning And Natural Algorithms Phd Thesis. with separate individual learning or local. Ant colony optimization algorithms have been applied to many combinatorial optimization problems. Learning and Natural Algorithms, PhD thesis. Optimization, Learning and Natural Algorithms.” blogger.comted Reading Time: 3 mins Ant Colony Optimization Utkarsh Jaiswal, Shweta Aggarwal Abstract-Ant colony optimization (ACO) is a new natural computation method from mimic the behaviors of ant colony. It is a very good combination optimization method. Ant colony optimization algorithm was recently proposed algorithm, it has strong robustness as well as
No comments:
Post a Comment